Artificial Intelligence

Artificial Intelligence (AI) has become increasingly popular in the last few years. As a result, it has become a part of everyday life and has been incorporated into many professional settings, including medicine and scientific research. While there has been a lot of “buzz” around AI, there remains to be confusion regarding what AI actually is.
The National Institute of Health defines AI as “a feature where machines learn to perform tasks, rather than simply carrying out computations that are input by human users”(1). So, this essentially means that AI is a computer or machine that has been programmed to “learn” how to perform a task on its own.This is done through machine learning which is when a computer “learns” how to perform a task using different algorithms and data sets (2). Deep learning is one type of machine learning that uses multi-layer computations to train computers on “large amounts of complex, unstructured data”(1). This is done to mimic intricate decision making done by humans (3).
We encounter AI on a daily basis like when we use a virtual assistant on our phone (Siri, Alexa, Google, etc.). Can you think of other examples where you encounter AI in your daily life?

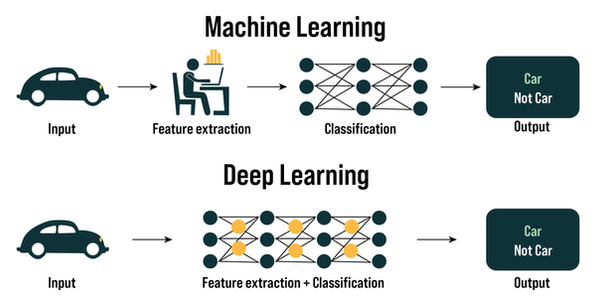
Machine learning requires more human intervention. Humans must provide data in a structured and clearly labelled manner for the learning model to execute the task (3).
Deep learning requires less intervention from humans. This is because a deep learning model can extract features and classify information from raw, unstructured data (3).
Through machine learning techniques, some types of AI can be:
-
Generative AI: when a computer is trained to generate new data, image, text, etc. (4).
-
Predictive AI: when a computer is trained to take in patterns in data to predict future outcomes (4).
With the rapid growth and incorporation of AI in day-to-day tasks, there has been a lot of debate on whether AI use is “good” or “bad.” In addition to that, how does AI affect science and scientific research, are there even any implications?
Before we jump into these discussions, what do you think?
Dangers of AI
With the rapid development of artificial intelligence, there are rising concerns about the dangers of AI. Below we have described four that have the potential to hinder research: ethics, lack of transparency, bias and discrimination, and misinformation and manipulation.
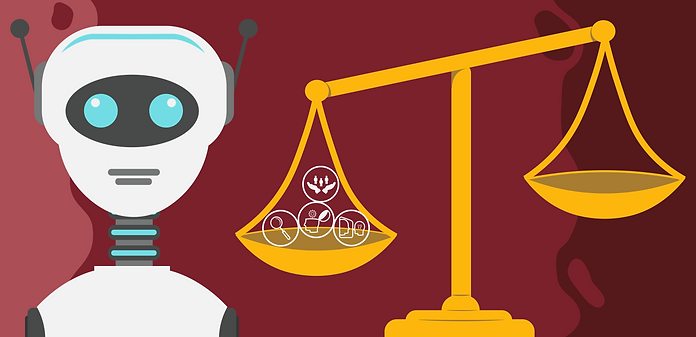
Ethics
It can be difficult to instill moral judgement and ethical values into an AI system through complex algorithms. Moreover, AI can be misused in several ways by humans in academic integrity, creativity, and decision-making (5, 6).
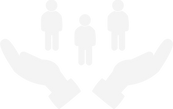
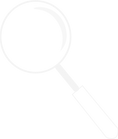
Lack of Transparency
Artificial Intelligence and deep learning models can be complex and hard to understand, and often present results and conclusions without describing the underlying processes. This can lead to both distrust of AI and resistance to using AI (5, 6).
Bias and Discrimination
AI systems have the ability to amplify biases and discriminations as they can carry forward the biases of the individuals who created the system/algorithm or through the training data sets (5, 6).
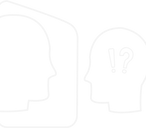
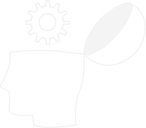
Misinformation and Manipulation
AI can generate various types of content, (for example, deepfakes), which can manipulate the truth and spread false information that can be easily trusted by the general public who might not have previous knowledge in the subject matter (6).
Benefits of AI
While it can be easy to immediately think of the negative implications of AI use in scientific research, AI also opens up a world of possibilities for scientists. This is especially true when thinking of lab work, drug discovery, and medicine.

Automation
AI can be used to automate certain parts of the experimental processes in the lab, saving scientists’ time so that they can focus on more complex problem solving. AI models can also be developed to direct robotic devices to complete experiments in the lab. A group in the University of Wisconsin is doing this to engineer proteins to make them capable of working at higher temperatures (which is usually not possible as high temperatures cause proteins to degrade) (7).
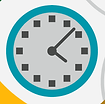

Drug Discovery
AI can help scientists predict whether potential new drugs will be successful or not. This is incredibly useful for drug discovery as it is usually a lengthy and costly process. Predicting the success of a potential new drug can save millions of dollars, save patients years of waiting for a cure, and ultimately lead to more effective drugs. AI models can be trained on previously discovered successful drugs to predict the likelihood that a potential new drug will be successful based on certain drug characteristics. Therefore, this can help researchers narrow down their pool of potential new drugs to follow up on and increase their chances of finding a successful drug (8, 9). Additionally, AI has also allowed scientists to find and create potential new drugs for testing that previously may not have been as quickly discovered (9, 10).
Medicine
AI can be used to analyze diagnostic images (such as MRI) to find finer details in an image which might be accidentally overlooked by a physician (1). AI can also make precision medicine more attainable. For example, the microbes in our gut play a huge role in contributing to disease progression from ailments like obesity to even Alzheimer’s. This is currently a growing area of research and medicine where an emphasis is being placed on having a healthy gut microbiome. Scientists are working on developing an AI model which can predict a patient’s biological age based on a fecal sample as well as provide diet plans to help patients maintain a healthy microbiome. This can revolutionize precision medicine (11).
* Look below for the definitions of the bolded words!

Click on each word to learn what it means...

Precision Medicine
“Innovative approach that uses information about an individual’s genomic, environmental, and lifestyle information to guide decisions related to their medical management” (12).
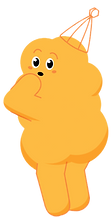
Hover over each box to see how AI is beneficial in the scenario
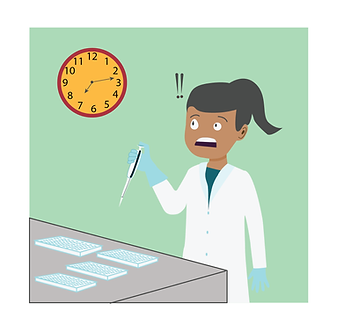

This scientist is stressed because they need create a presentation summarizing all their recent findings AND finish running all of these experiments!
Now, the scientist can create the presentation while this machine is programmed to transfer all the liquids necessary to complete the experiment!
This scientist is working on developing new antibiotic drugs, but is having trouble narrowing down the list of chemicals to test in the lab.
By using a trained AI model, the model can predict which chemicals are most suitable as candidates for new antibiotics. With this information, the scientist can test these chemicals in the lab!
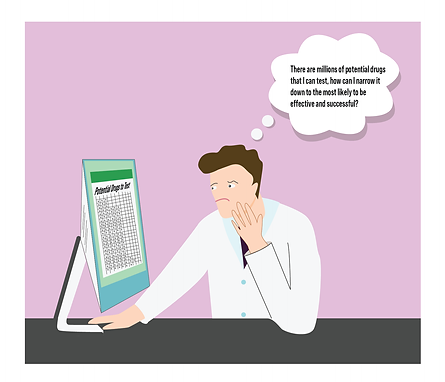
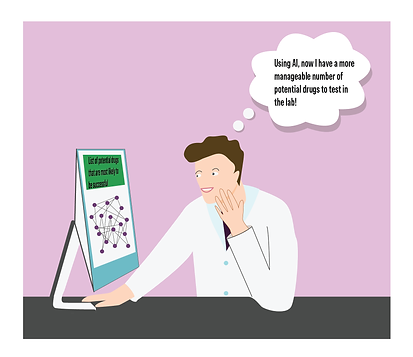
Now, have you changed your mind about AI?
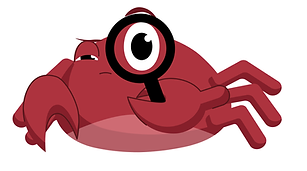
To avoid using AI in a harmful way, it is important to:
-
Develop unbiased algorithms and diverse training data sets (5).
-
Develop ethical practices for engaging with AI (6).
-
Be transparent about the use of AI within your work (14).
While AI has the potential to harm research processes and results, it is equally crucial to consider the benefits it has to offer. There is no clear dichotomy on whether AI is “good” or “bad.” One must weigh the pros and cons and plan the use of AI to determine one’s stance.
References
-
“Artificial Intelligence (AI).” National Institute of Biomedical Imaging and Bioengineering. February, 2020. https://www.nibib.nih.gov/sites/default/files/2022-05/Fact-Sheet-Artifical-Intelligence.pdf
-
“What is machine learning (ML)?” IBM. Accessed April 15, 2024, https://www.ibm.com/topics/machine-learning.
-
“What is deep learning?” IBM. Accessed April 15, 2024, https://www.ibm.com/topics/deep-learning#:~:text=Deep%20learning%20is%20a%20subset,AI)%20in%20our%20lives%20today.
-
Siegel, Eric. “3 Ways Predictive AI Delivers More Value Than Generative AI.” Forbes. March 4, 2024. https://www.forbes.com/sites/ericsiegel/2024/03/04/3-ways-predictive-ai-delivers-more-value-than-generative-ai/?sh=4a7e61754e84.
-
Thomas, Mike. “12 Risks and Dangers of Artificial Intelligence (AI).” builtin. Last modified March 1, 2024. https://builtin.com/artificial-intelligence/risks-of-artificial-intelligence.
-
Marr, Bernard. “The 15 Biggest Risks Of Artificial Intelligence.” Forbes. June 2, 2023. https://www.forbes.com/sites/bernardmarr/2023/06/02/the-15-biggest-risks-of-artificial-intelligence/?sh=594c22952706.
-
Callaway, Ewen. “‘Set It and Forget It’: Automated Lab Uses AI and Robotics to Improve Proteins.” Nature. January 11, 2024. https://www.nature.com/articles/d41586-024-00093-w.
-
Mock, Marissa, Suzanne Edavettal, Christopher Langmead and Alan Russell. “AI Can Help to Speed Up Drug Discovery — But Only If We Give It the Right Data.” Nature. September 11, 2023. https://www.nature.com/articles/d41586-023-02896-9#:~:text=Biopharmaceutical%20companies%20are%20now%20using,and%20about%20properties%20of%20interest.
-
Swanson, Kyle, Gary Liu, Denise B. Catacutan, Autumn Arnold, James Zou and Jonathan M. Stokes. “Generative AI for Designing and Validating Easily Synthesizable and Structurally Novel Antibiotics.” Nature Machine Intelligence 6, (2024): 338–353, doi: 10.1038/s42256-024-00809-7.
-
Heaven, Will Douglas. “AI is Dreaming Up Drugs That No One Has Ever Seen. Now We’ve Got To See If They Work.” MIT Technology Review. February 15, 2023. https://www.technologyreview.com/2023/02/15/1067904/ai-automation-drug-development/.
-
“Unravelling Complex Biology Through Big Data and AI.” The Uehara Memorial Foundation. Accessed April 15, 2024, https://www.nature.com/articles/d42473-023-00211-8.
-
Delpierre, Cyrille and Thomas Lefèvre. “Precision and Personalized Medicine: What their Current Definition Says and Silences About the Model Of Health they Promote. Implication for the Development of Personalized Health.” Frontiers in Sociology 8, (2023): 1112159, doi: 10.3389/fsoc.2023.1112159.
-
“Microbiome.” National Institute of Environmental Health Sciences. Last modified March 22, 2024.
-
Neeley, Tsedal. “8 Questions About Using AI Responsibly, Answered.” Harvard Business Review. May 9, 2023. https://hbr.org/2023/05/8-questions-about-using-ai-responsibly-answered.
Authors and designers of this learning module: Dana Kukje Zada and Kavya Patel
About Us
Science for Everyone is a Canadian Nonprofit Organization that provides educational resources to help raise the level of scientific literacy in the general population.